Part 4 of: The Decentralized Cybersecurity Paradigm: Rethinking Traditional Models
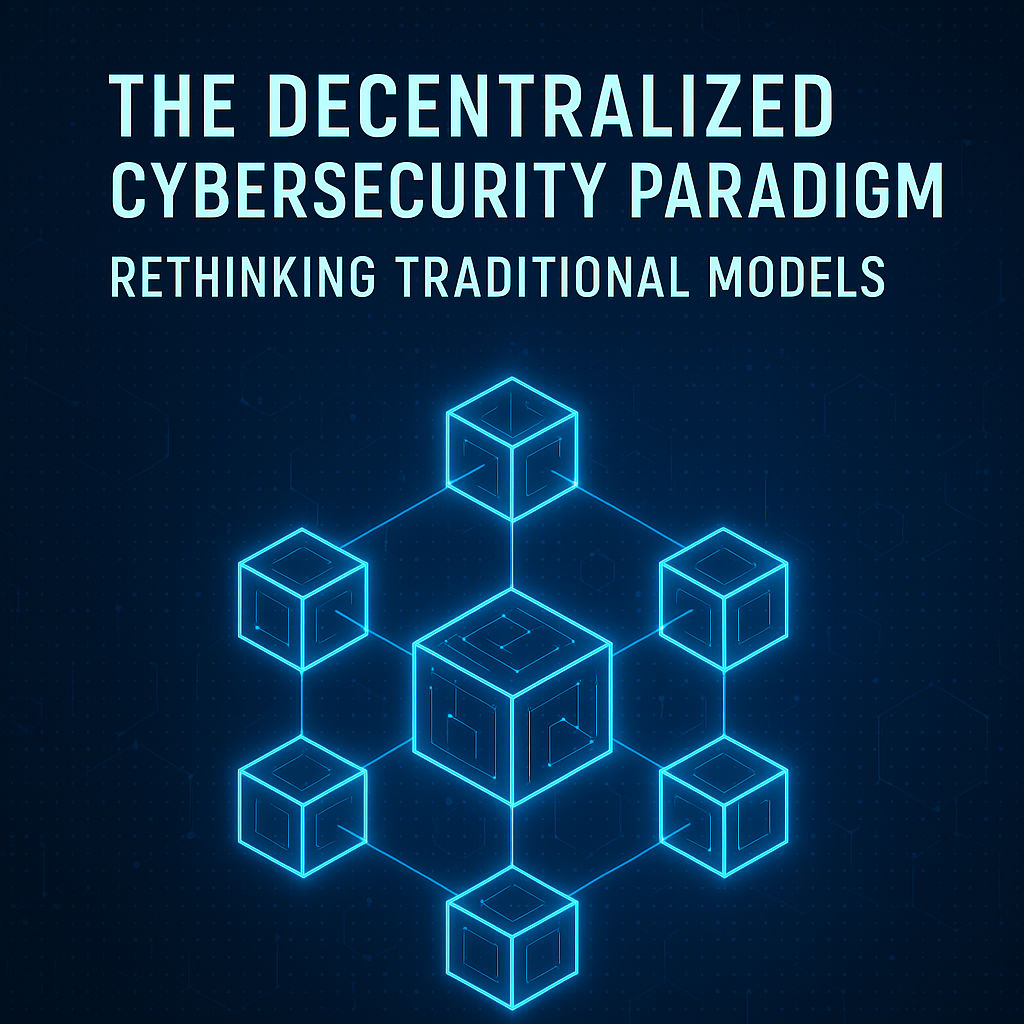
In Part 3 we reviewed the role of zero-knowledge proofs in enhancing data security. Decentralization has potential in multiple areas, in particular anti-fragility through decentralized security systems.
The digital landscape is facing an escalating barrage of sophisticated and frequent cyberattacks. This makes for obvious challenges. Traditional centralized security models serve as the old guard of cybersecurity at this point. These models ruled for decades and are now revealing their limitations in the face of evolving threats. Centralized systems concentrate power and control within a single entity. This setup creates a tempting and rewarding target for malicious actors. Storing data, enforcing security, and making decisions in one place increases risk. A successful breach can expose massive amounts of data. It can also disrupt essential services across the entire network. Moreover, as ecosystems are now more complex. Cloud computing, IoT, and remote work have changed the security landscape. These developments challenge centralized solutions to provide adequate coverage. They also strain flexibility and scalability in traditional security architectures.
In response to these challenges, forward thinking cybersecurity leaders are shifting towards decentralized cybersecurity. These paths offer much promise in building more resilient and fault-tolerant security systems. Decentralization, at its core, involves distributing power and control across multiple independent points within an ecosystem, rather than relying on a single central authority (https://artem-galimzyanov.medium.com/why-decentralization-matters-building-resilient-and-secure-systems-891a0ba08c2d). This shift in architectural philosophy is fundamental. It can greatly improve a system’s resilience to adverse events. Even if individual components fail, the system can continue functioning correctlys (https://www.owlexplains.com/en/articles/decentralization-a-matter-of-computer-science-not-evasion/).
Defining Resilience and Fault Tolerance in Cybersecurity
To understand how decentralized principles enhance security, it is crucial to first define the core concepts of resilience and fault tolerance within the cybersecurity context.
Cyber Resilience
The National Institute of Standards and Technology (NIST) defines cyber resilience as the ability to anticipate, withstand, recover from, and adapt to cyber-related disruptions (https://www.pnnl.gov/explainer-articles/cyber-resilience). Cyber resilience goes beyond attack prevention, it ensures systems remain functional during and after adverse cyber events. A cyber-resilient system anticipates threats, resists attacks, recovers efficiently, and adapts to new threat conditions. This approach accepts breaches as inevitable and focuses on maintaining operational continuity. Cyber resilience emphasizes the ability to quickly restore normal operations after a cyber incident.
Fault Tolerance
Fault tolerance refers to the ability of a system to continue operating correctly even when one or more of its components fail (https://www.zenarmor.com/docs/network-security-tutorials/what-is-fault-tolerance). The primary objective of fault tolerance is to prevent disruptions arising from Single Points Of Failure (SPOF). Fault-tolerant systems use backups like redundant hardware and software to maintain service during component failures. These backups activate automatically to ensure uninterrupted service and high availability when issues arise. Fault tolerance ensures systems keep running seamlessly despite individual component failures. Unlike resilience, fault tolerance focuses on immediate continuity rather than long-term adaptability. Resilience addresses system-wide adversity; fault tolerance handles localized, real-time malfunctions.
Both resilience and fault tolerance are critically important for modern security systems due to the increasing volume and sophistication of cyber threats. The interconnected and complex nature of today’s digital infrastructure amplifies the potential for both targeted attacks and accidental failures. A strong security strategy uses layers: prevention, response, recovery, and continued operation despite failures. It combines proactive defenses with reactive capabilities to handle incidents and withstand attacks. Effective incident management ensures rapid recovery after cyber events. Systems must function even when components or services fail. This approach maintains uptime, safeguards data integrity, and preserves user trust against evolving threats.
The Case for Decentralization: Enhancing Security Through Distribution
Traditional centralized security systems rely on a single control point and central data storage. This centralized design introduces critical limitations that increase vulnerability to modern cyber threats. By concentrating power and data in one place, these systems attract attackers. A single successful breach can trigger widespread and catastrophic damage. Centralization also creates bottlenecks in incident management and slows down mitigation efforts.
Decentralized security systems offer key advantages over centralized approaches. They distribute control and decision-making across multiple independent nodes. This distribution removes SPOF and enhances fault tolerance. Decentralized systems also increase resilience across the network. Attackers must compromise many nodes to achieve meaningful disruption.
Decentralized security enables faster, localized responses to threats. Each segment can tailor its defense to its own needs. While decentralization may expand the attack surface, it also complicates large-scale compromise. Attackers must exert more effort to breach multiple nodes. This effort is far greater than exploiting one weak point in a centralized system.
Decentralization shifts risk from catastrophic failure to smaller, isolated disruptions. This model significantly strengthens overall security resilience.
Key Decentralized Principles for Resilient and Fault-Tolerant Security
Several key decentralized principles contribute to the creation of more resilient and fault-tolerant security systems. These principles, when implemented effectively, can significantly enhance an organization’s ability to withstand and recover from cyber threats and system failures.
Distribution of Components and Data
Distributing security components and data across multiple nodes is a fundamental aspect of building resilient systems (https://www.computer.org/publications/tech-news/trends/ai-ensuring-distributed-system-reliability/). The approach is relatively straightforward. The aim is that if one component fails or data is lost at one location, other distributed components or data copies can continue to provide the necessary functions. By isolating issues and preventing a fault in one area from spreading to the entire system, distribution creates inherent redundancy. This directly contributes to both fault tolerance and resilience. For instance, a decentralized firewall ecosystem can distribute its rulesets and inspection capabilities across numerous network devices. This ensures that a failure in one device does not leave the entire network unprotected. Similarly, distributing security logs across multiple storage locations makes it significantly harder for an attacker to tamper with or delete evidence of their activity.
Leveraging Redundancy and Replication
Redundancy and replication are essential techniques for achieving both fault tolerance and resilience. Redundancy involves creating duplicate systems, both hardware and software, to provide a functional replica that can handle production traffic and operations in case of primary system failures. Replication, on the other hand, focuses on creating multiple synchronized copies, typically of data, to ensure its availability and prevent loss.
Various types of redundancy can be implemented, including hardware redundancy (duplicating physical components like servers or network devices), software redundancy (having backup software solutions or failover applications), network redundancy (ensuring multiple communication paths exist), and data redundancy (maintaining multiple copies of critical data). Putting cost aside for the moment, the proliferation of cloud technologies has made this achievable to any and all willing to put some effort into making systems redundant. Taking this a step further, these technologies make it entirely possible to push into the high availability state of resilience. Here failover is seamless. By having running replicas readily available, a system can seamlessly switch over from a filed instance to a working component or better yet route live traffic to pursue high availability at run time. This requires proper architecting and that budget we put aside earlier.
The Power of Distributed Consensus
Distributed consensus mechanisms play a crucial role in building trust and ensuring the integrity of decentralized security systems (https://medium.com/@mani.saksham12/raft-and-paxos-consensus-algorithms-for-distributed-systems-138cd7c2d35a). These mechanisms enable state agreement amongst multiple nodes, even when some nodes might be faulty or malicious. Algorithms such as Paxos, Raft, and Byzantine Fault Tolerance (BFT) are designed to achieve consensus in distributed environments, ensuring data consistency and preventing unauthorized modifications. In a decentralized security context, distributed consensus ensures that security policies and critical decisions are validated by a majority of the network participants. This increases the system’s resilience against tampering and SPOF.
For example, Certificate Transparency (CT) serves as a real-world application of this technology used to combat the risk of maliciously issued website certificates. Instead of relying solely on centralized Certificate Authorities (CAs), CT employs a system of public, append-only logs that record all issued TLS certificates using cryptographic Merkle Trees. Multiple independent nodes monitor and constantly observe these logs, verifying their consistency and detecting any unlogged or suspicious certificates. Web browsers enforce CT by requiring certificates to have a Signed Certificate Timestamp (SCT) from a trusted log. This requirement effectively creates a distributed consensus among logs, monitors, auditors, and browsers regarding the set of valid, publicly known certificates and making it significantly harder for certificate tampering.
Enabling Autonomous Operation
Decentralized security systems can leverage autonomous operation to enhance the speed and efficiency of security responses (https://en.wikipedia.org/wiki/Decentralized_autonomous_organization). Decentralized Autonomous Organizations (DAOs) and smart contracts can automate security functions, such as updating policies or managing access control, based on predefined rules without any human intervention. Furthermore, autonomous agents can be deployed in a decentralized manner to do things such as continuously monitor network traffic, detect anomalies, detect threats, and respond in real-time without the need for manual intervention. This capability allows for faster reaction times to security incidents. Moreover, it improves the system’s ability to adapt to dynamic and evolving threats.
Implementing Self-Healing Mechanisms
Self-healing mechanisms are a vital aspect of building resilient decentralized security systems. These mechanisms enable an ecosystem to automatically detect failures or intrusions and initiate recovery processes without human intervention. Techniques such as anomaly detection, automated recovery procedures, and predictive maintenance can be employed to ensure that a system can adapt to and recover from incidents with minimal downtime (https://www.computer.org/publications/tech-news/trends/ai-ensuring-distributed-system-reliability/). For example, if a node in a decentralized network is compromised, a self-healing mechanism could automatically isolate that affected node, restore its functionality to a new node (from a backup), and/or reallocate its workload to the new restored node or to other healthy nodes in the network.
Algorithmic Diversity
Employing algorithmic diversity in decentralized security systems can significantly enhance their resilience against sophisticated attacks. This principle involves using multiple different algorithms to perform the same security function. For example, a decentralized firewall might use several different packet inspection engines based on varying algorithms. This diversity makes it considerably harder for attackers to enumerate and/or fingerprint entities or exploit a single vulnerability to compromise an entire system. Different algorithms simply have distinct weaknesses and so diversity in this sense introduces resilience against systemic impact (https://www.es.mdh.se/pdf_publications/2118.pdf). By introducing redundancy at the functional level, algorithmic diversity strengthens a system’s ability to withstand attacks that specifically target algorithmic weaknesses.
Applications of Decentralized Principles in Security Systems
The decentralized principles discussed so far in this series can be applied to various security systems. The goal is to enhance their resilience and fault tolerance. Here are some specific examples:
- Decentralized Firewalls
- Robust Intrusion Detection and Prevention Systems
- Decentralized Key Management
Decentralized Firewalls
Traditional firewalls, operating as centralized or even standalone appliances, can become bottlenecks and/or SPOF in modern distributed networks. Decentralized firewalls offer a more robust alternative by embedding security services directly into the network fabric (https://www.paloaltonetworks.com/cyberpedia/what-is-a-distributed-firewall). These firewalls distribute their functionalities across multiple points within a network. This is often as software agents running on individual hosts or virtual instances. This distributed approach provides several advantages, including enhanced scalability to accommodate evolving and/or growing networks, granular policy enforcement tailored to specific network segments, and improved resilience against network failures as the security perimeter is no longer reliant on a single device. Decentralized firewalls can also facilitate micro-segmentation. This allows for precise control over traffic flow and potentially limits the lateral movement of attackers within the network.
Building Robust Intrusion Detection and Prevention Systems (IDS/IPS)
Intrusion Detection Systems (IDS) and Intrusion Prevention Systems (IPS) can benefit significantly from decentralized principles. Instead of relying on a centralized system to monitor and analyze network traffic, a decentralized IDS/IPS involves deploying multiple monitoring and analysis units across a network. This distributed architecture offers improved detection capabilities for distributed attacks, enhanced scalability to cover large networks, and increased resilience against SPOF. Furthermore, decentralized IDS/IPS can leverage federated learning techniques, allowing multiple devices to train detection models without the need to centralize potentially sensitive data.
Decentralized Key Management
Managing cryptographic keys in a decentralized manner has potential for securing sensitive data. Traditional centralized key management systems present a SPOF. If compromised, these could needlessly expose a lot of data. Decentralized Key Management Systems (DKMS) address this issue by distributing the control and storage of cryptographic keys across multiple network locations or entities. Techniques such as threshold cryptography, where a secret key is split into multiple shares, and distributed key generation (DKG) ensure that no single party holds the entire key, making it significantly harder for attackers to gain unauthorized access. Technologies like blockchains can also play a role in DKMS. They provide a secure, transparent, and auditable platform for managing and verifying distributed keys.
Blockchain Technology: A Cornerstone of Resilient Decentralized Security
Blockchain technology, with its inherent properties of decentralization, immutability, and transparency, serves as a powerful cornerstone for building resilient decentralized security systems. In particular, blockchain is ideally suited for ensuring the integrity and trustworthiness of elements such as logs. The decentralized nature of blockchain means that elements such as security logs can be distributed across multiple nodes. This makes it virtually impossible for a single attacker to tamper with or delete any of that log data without the consensus of the entire network. An attacker trying to clean their tracks via wiping or altering log data would not be successful if log data was handled in such a way.
The cryptographic hashing and linking of blocks in a blockchain create an immutable record of all events. This provides enhanced data integrity and non-repudiation. This tamper-proof audit trail is invaluable for cybersecurity forensics, incident response, and demonstrating compliance with regulatory requirements. While blockchain offers apparent security benefits for logging, its scalability can be a concern for high-volume logging scenarios. Solutions such as off-chain storage with on-chain hashing or specialized blockchain architectures are being explored to address these limitations (https://hedera.com/learning/distributed-ledger-technologies/blockchain-scalability).
Advantages of Decentralized Security
Embracing decentralized principles for security offers multiple advantages that contribute to building more resilient and fault-tolerant systems. By distributing control and resources, these systems inherently avoid any SPOF. These are of course a major vulnerability in centralized architectures. The redundancy and replication inherent in decentralized designs significantly improve fault tolerance, ensuring that a system can continue operations even if individual components fail. The distributed nature of these types of systems also enhances security against attacks. Nefarious actors would need to compromise many disparate parts of a network to achieve their objectives.
Decentralized principles, particularly when combined with blockchain technology, can lead to enhanced data integrity and trust. The mechanisms allowing this are distributed consensus and immutable record-keeping (https://www.rapidinnovation.io/post/the-benefits-of-decentralized-systems). In many cases, decentralization can empower users with greater control over their data and enhance privacy. Depending on the specific implementation, decentralized systems can also offer improved scalability and performance, especially for distributed workloads. Finally, the distributed monitoring and autonomous operation often found in decentralized security architectures can lead to faster detection and response to threats, boosting overall resilience.
Challenges of Decentralized Security
Despite the numerous advantages, implementing decentralized security systems also involves navigating several challenges and considerations. The architecture, design, and management of distributed systems can be inherently more complex than traditional centralized models. They require specialized expertise and careful architectural planning. The distributed nature of these systems can also introduce potential performance overhead due to the need for consensus among multiple nodes. This also creates conditions of increased communication chatter across a network. Further complications can be encountered when troubleshooting issues as those exercises are no longer straightforward.
Ensuring consistent policy enforcement across a decentralized environment can also be challenging. This requires robust mechanisms for policy distribution and validation. Furthermore, there is an increased attack surface presented by a larger number of network nodes. This is natural in highly distributed systems and it necessitates meticulous management and security controls to prevent vulnerabilities from being exploited.
Organizations looking to adopt decentralized security must also carefully consider regulatory and compliance requirements. These might differ for distributed architectures compared to traditional centralized systems. Robust key management strategies are paramount in decentralized environments to secure cryptographic keys distributed across multiple entities. Finally, effective monitoring and incident response mechanisms need to be adapted for the distributed nature of these systems to ensure timely detection and mitigation of incidents.
Real-World Examples
Blockchain-based platforms like Hyperledger Indy and ION are enabling decentralized identity management. This gives users greater control over their digital identities while enhancing security and privacy (https://andresandreu.tech/the-decentralized-cybersecurity-paradigm-rethinking-traditional-models-decentralized-identifiers-and-its-impact-on-privacy-and-security/). Decentralized data storage solutions such as Filecoin and Storj leverage distributed networks to provide secure and resilient data storage, eliminating SPOF. BlockFW demonstrates the potential of blockchain for creating rule-sharing firewalls with distributed validation and monitoring. These examples highlight the growing adoption of decentralized security across various sectors. They also demonstrate practical value in addressing the limitations of traditional centralized models.
Ultimately, embracing decentralized principles offers a pathway towards building more resilient and fault-tolerant security systems. By distributing control, data, and security functions across multiple network nodes, organizations can overcome the inherent limitations of centralized architectures, mitigating the risks associated with SPOF and enhancing their ability to withstand and recover from cyber threats and system failures. The key decentralized principles of distribution, redundancy, distributed consensus, autonomous operations, and algorithmic diversity contribute uniquely to a more robust and adaptable security posture.
Blockchain technology stands out as a powerful enabler of decentralized security. While implementing decentralized security systems presents certain challenges related to complexity, management, and performance, the advantages in terms of enhanced resilience, fault tolerance, and overall security are increasingly critical in today’s continuously evolving threat landscapes. As decentralized technologies continue to mature and find wider adoption, they hold significant power in reshaping the future of cybersecurity.
In Part 5 of this decentralized journey we will further explore some of the challenges and opportunities of decentralized security in enterprises.