Part 4 in the Series: Unlock Artificial Intelligence Potential – The Power Of Pristine Data
The integration of Artificial Intelligence (AI) into cybersecurity has ushered in a new era of sophisticated threat detection, proactive vulnerability assessments, and automated incident response. As organizations increasingly rely on AI to bolster their defenses, the fundamental principle remains that the quality of the data on which they train these advanced systems directly links to their effectiveness. The old saying “garbage in, garbage out” (GIGO) holds true here; to avoid a data fidelity crisis: secure AI now before cybersecurity fails.
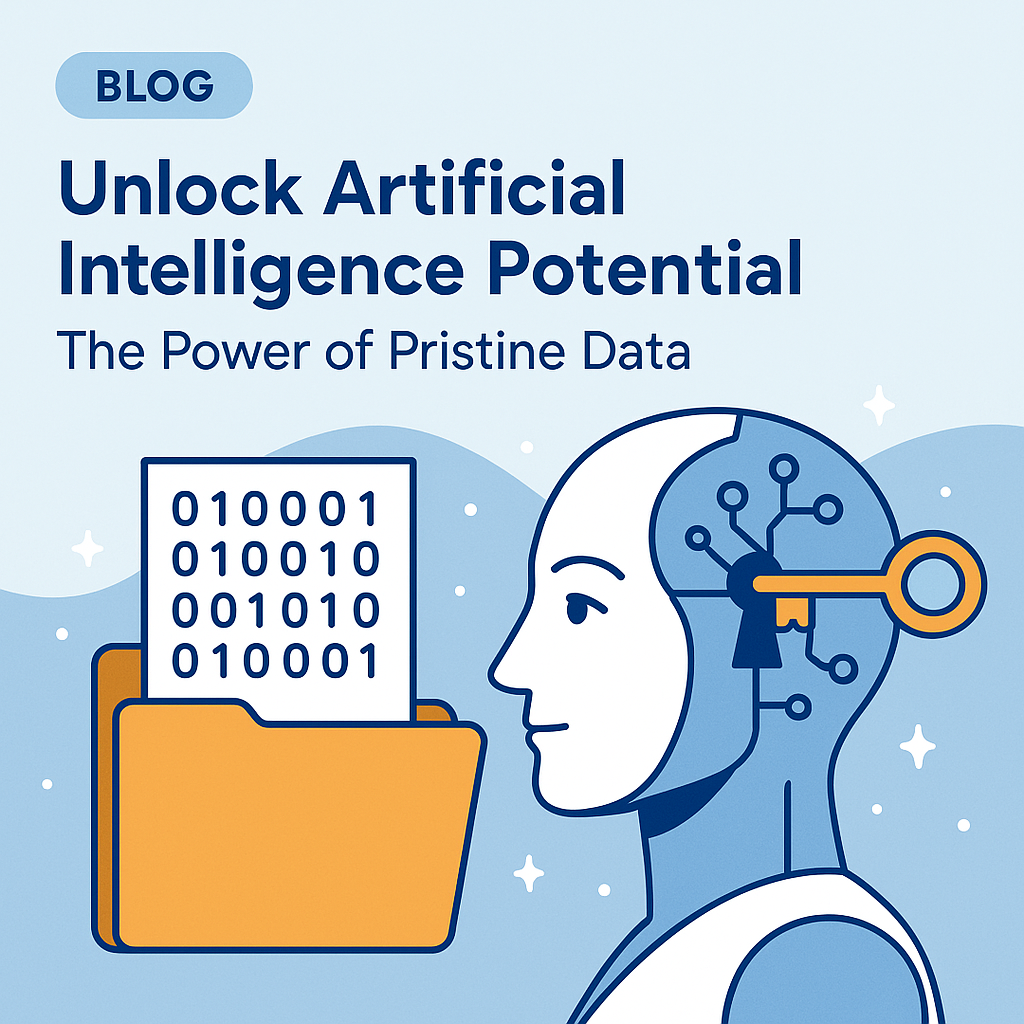
In Part 3 we covered some examples of cybersecurity AI applications and how they could be negatively impacted. Beyond the general cleanliness of data, the fidelity, or the accuracy and faithfulness of the data to its source, plays a crucial role in ensuring the trustworthiness of AI applications in cybersecurity.
Inaccurate Data
The use of inaccurate data in training AI models can have profound negative consequences. It can lead to flawed outcomes, resulting in significant repercussions for organizations relying on these systems for security. For instance, take an active protection system designed for an Industrial Control Systems (ICS) environment. Protection can be based on set point values that mean physical changes to some equipment. These set point values typically exist in some range for normal operational functions. A model can be trained with inaccurate data values that are outside the range of normal operational parameters. This means bad data may get past the active protection system. This in turn could have a potential physical impact.
Biased Data
Inaccurate or unreliable AI results can erode user trust and confidence in an entire AI system. Users can become hesitant to rely on its outputs for critical security decisions. Biased training data is another significant concern that can compromise the fidelity of AI models. AI models learn from the patterns present in their training data. If this data reflects existing societal, historical, or systemic biases, the AI model will likely inherit and operationalize those biases (https://versium.com/blog/ais-achilles-heel-the-consequence-of-bad-data). In cybersecurity, this can lead to the development of unfair or ineffective security measures. These can take the form of AI systems that disproportionately flag activities from certain user groups or source countries as suspicious.
Biased data can also result in AI models that perform poorly. This can manifest as an increased rate of false positives or false negatives for specific demographics. In turn, this can skew the overall fairness and effectiveness of a security system (https://interface.media/blog/2024/12/24/exploring-the-impact-of-ai-bias-on-cybersecurity/).
Poisoned Data
One of the most concerning threats to data fidelity in AI is the risk of manipulated or poisoned data. Data poisoning is when malicious actors intentionally introduce false or misleading data into some training process. This is done to either degrade the AI model’s performance or to cause it to behave in a way that benefits the attacker (https://akitra.com/cybersecurity-implications-of-data-poisoning-in-ai-models/). These types of attacks can be very difficult to detect. Especially if there is a lack of intimacy with the original unpoisoned data set. These types of attacks can lead to compromised security postures where AI models put cybersecurity resources into time suck scenarios, fail to detect real threats, or flag legitimate actions as suspicious. Model poisoning can also result in biased outcomes, provide unauthorized access to systems, or cause a disruption of critical services.
A related threat is that of adversarial attacks (e.g. Adversarial AI). This is where subtle modifications are made to the input data at the time of inference to intentionally fool an AI model into making incorrect classifications or decisions. In the context of cybersecurity, this could involve attackers subtly altering malware signatures to evade detection by AI-powered antivirus systems. Another example is the alteration of AI managed Web Application Firewall (WAF) rulesets and/or regular expressions.
The integrity of training data is therefore paramount. Biases can lead to systemic flaws in how security is applied. Intentional manipulation can directly undermine the AI’s ability to function correctly. This creates potential new attack surface elements where none previously existed.
Part 5 will cover some technical insights to unlock artificial intelligence potential and avoid a data fidelity crisis: secure AI now before cybersecurity fails.