Part 3 in the series -Unlock Artificial Intelligence Potential – The Power Of Pristine Data
The integration of Artificial Intelligence (AI) into cybersecurity has ushered in a new era of sophisticated threat detection, proactive vulnerability assessments, and automated incident response. As organizations increasingly rely on AI to bolster their defenses, the fundamental principle remains that the quality of the data on which they train these advanced systems directly links to their effectiveness. The old saying “garbage in, garbage out” (GIGO) holds true here; bad data is undermining your cybersecurity AI right now.
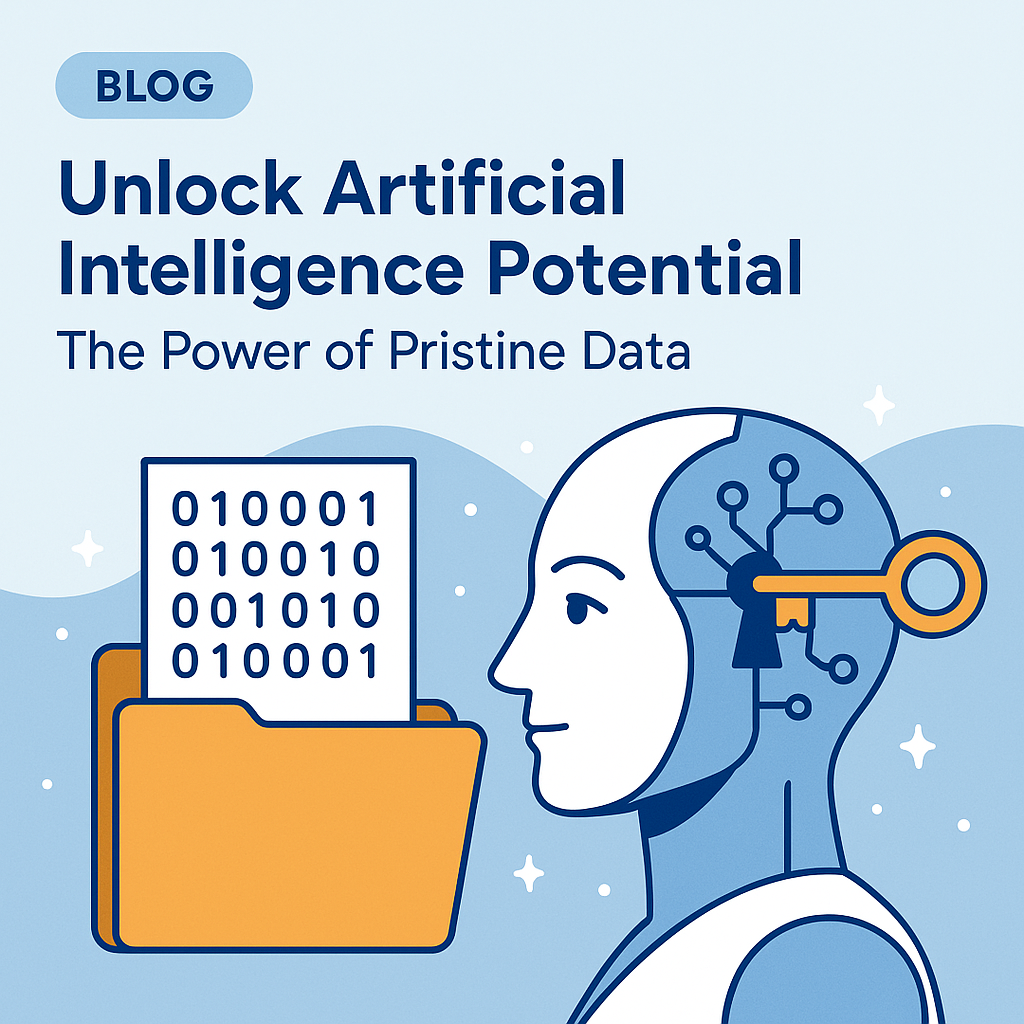
In Part 2 we covered the perils of poor data hygiene. The promise of AI in cybersecurity lies in its ability to outperform a group of qualified humans. From the defenders perspective, this generally equates to:
- analyzing vast amounts of data
- identifying subtle patterns
- responding to threats at speeds that are impossible for those human analysts
Like so many things in AI, the effectiveness of these applications heavily depends on the quality of the data used to train them. Poor data hygiene can severely cripple the performance of AI in critical cybersecurity tasks.
Threat Detection
Once everyone gets past the hype, threat Detection is a major area where cybersecurity practitioners are expecting much benefit. AI has shown immense potential, excelling at identifying patterns and anomalies in real-time to flag potential security threats. However, the presence of poor data hygiene can significantly undermine this capability.
Missed threats, also known as false negatives, represent a major area of impact. The formula here is relatively straightforward: AI models require data to understand what constitutes a threat. If that training data is incomplete or if it lacks examples of new and evolving attack patterns, the AI might fail to recognize novel threats when they first appear in the real world. Biased data can also lead to AI engines overlooking certain attacks, potentially creating blind spots in a security ecosystem (https://www.researchgate.net/publication/387326774_Effect_of_AI_Algorithm_Bias_on_the_Accuracy_of_Cybersecurity_Threat_Detection_AUTHORS).
On the other end of the spectrum are false positives. Poor data hygiene can lead to these phenomena where AI incorrectly flags benign activities as malicious. This can be caused by noisy or inconsistent data that confuses a model, leading it to misinterpret normal behavior as suspicious. The consequence of excessive false positives is often white noise and alert fatigue among security teams. The constant stream of non-genuine alerts can cause analysts to become desensitized. The risk is then potentially missing actual threats that in some cases are blatantly obvious.
Bias in the training data can also result in a reduced ability to detect novel threats. This can lead to inaccurate assessments, causing a misprioritization of security efforts. The effectiveness of AI in threat detection fundamentally depends on the diversity and representativeness of the training data. If the data does not cover the full spectrum of attack types and normal network behaviors, the AI will struggle to accurately distinguish between them.
Vulnerability Assessment
Another critical cybersecurity function increasingly employing AI is vulnerability assessment, where AI continuously scans systems (networks, applications, APIs, etc.) for weaknesses and prioritizes them based on potential impact. Organizations highly value this capability because human resources cannot keep pace with the volume of findings in larger environments. Business context plays a huge role here. It becomes the driver for what is a priority to a given organization. Business context would therefore be a data set used to train models for the purpose of vulnerability assessments.
Inaccurate data can severely hinder the reliability of AI in this area. Inaccurate data can severely hinder the reliability of AI in this area. Incomplete or incorrect training data, or mislabeling assets, may cause AI to miss or misprioritize vulnerabilities. This could leave systems exposed to potential exploitation. Conversely, inaccurate data could also lead to the AI incorrectly flagging non-existent vulnerabilities or treating assets of non-value as critical, wasting resources on addressing threats incorrectly.
Biased or outdated data can also result in an inaccurate prioritization of vulnerabilities. This can lead to a misallocation of security resources towards less critical issues while more severe weaknesses remain unaddressed. Ultimately, poor data hygiene can lead to a compromised security posture due to unreliable assessments of the true risks faced by an organization. AI’s ability to effectively assess vulnerabilities depends on having precise and current information about the business and vulnerabilities themselves. Inaccuracies in this foundational data can lead to a false sense of security or the inefficient deployment of resources to address vulnerabilities that pose minimal actual risk.
Phishing Detection
The detection of phishing activity has seen significant advancements through the application of AI. This is particularly so with the use of Natural Language Processing (NLP). NLP allows AI to analyze email content, discover sentiment, identify sender behavior, and use contextual information to identify and flag potentially malicious messages. Despite its successes, the effectiveness of AI in phishing detection is highly sensitive to poor data hygiene.
One significant challenge is the failure to detect sophisticated attacks. If the training data used to teach the AI what constitutes a phishing email lacks examples of the latest and most advanced phishing techniques the AI might not be able to recognize these new threats. The scenario of AI vs AI is becoming a reality in the realm of phishing detection. The defensive side is up against those leveraging generative AI to create highly realistic, strategic, and personalized messages. This is particularly concerning as phishing tactics are becoming very realistic and they are constantly evolving to evade detection.
Inconsistent or noisy data within email content or sender information can lead to an increase in false positives. Legitimate emails could get incorrectly flagged as phishing attempts. This can disrupt communication and lead to user frustration. Bias in training data can cause AI to miss phishing attacks targeting certain demographics or generate excessive false positives. Given the ever-changing nature of phishing attacks, it is crucial for AI models to be continuously trained on diverse and up-to-date datasets that include examples of the most recent and sophisticated tactics employed by cybercriminals. Poor data hygiene can leave the AI unprepared and ineffective against these evolving threats.
Part 4 will cover the significance of data fidelity and how the lack of trustworthiness can negatively impact an environment. Bad data is undermining your cybersecurity AI right now.