Part 7 in the Series: Unlock Artificial Intelligence Potential – The Power Of Pristine Data
The integration of Artificial Intelligence (AI) into cybersecurity has ushered in a new era of sophisticated threat detection, proactive vulnerability assessments, and automated incident response. As organizations increasingly rely on AI to bolster their defenses, the fundamental principle remains that the quality of the data on which they train these advanced systems directly links to their effectiveness. The old saying “garbage in, garbage out” (GIGO) holds true here; sometimes it is done correctly, data-powered AI: proven cybersecurity examples you need to see.
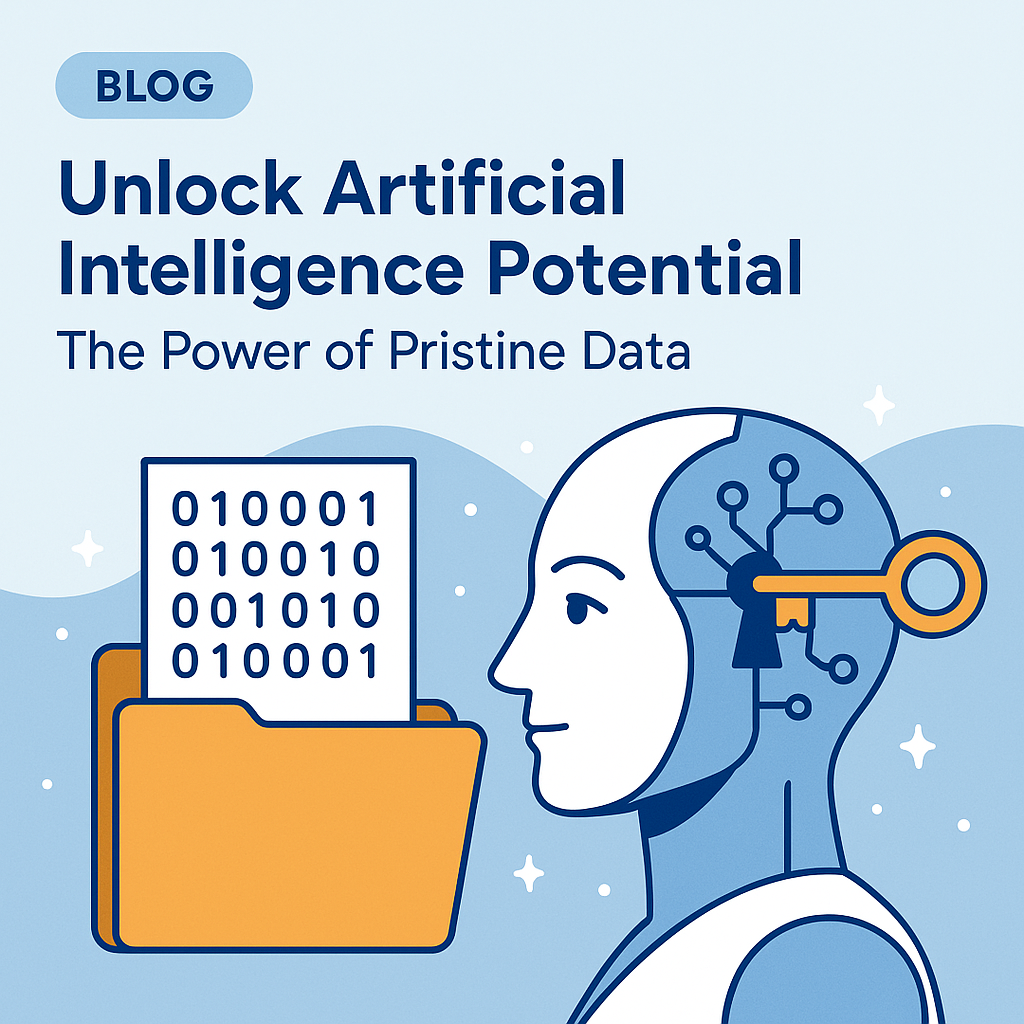
In Part 6 we covered some data hygiene secrets, or best practices. These can prove to be fundamental to the quality of data used for training models. The importance of high-quality data in AI-powered cybersecurity is underscored by numerous real-world examples of systems that have demonstrated remarkable effectiveness.
Some Examples
Darktrace stands out as a pioneer in using AI for threat detection. To begin with, its system operates by learning the normal behavior patterns of users, devices, and networks within an organization. Once established, it identifies outliers—deviations that may indicate a cyber threat. Moreover, Darktrace analyzes network and user data in real time, helping prevent cyberattacks across multiple industries. For example, it detected and responded to a ransomware attack at a healthcare organization before the attacker could encrypt critical data. Ultimately, this success hinges on its ability to learn a highly accurate baseline of normal behavior. To achieve this, it requires a continuous stream of clean and representative data.
Constella Intelligence has also demonstrated the power of high-quality data in AI-driven cybersecurity. At the core of their approach, Constella’s solutions focus on identity risk management and threat intelligence, leveraging a vast data lake of curated and verified compromised identity assets. In a notable example, a top global bank used Constella to identify a threat actor and uncover a broader group selling stolen credentials. As a result, Constella’s AI helped stop fraud, saving the bank over $100 million by preventing massive credit card abuse. In addition, Constella’s “Hunter” platform—built on this rich data foundation—has been successfully used by cybercrime investigative journalist Brian Krebs to track and identify key figures in the cybercriminal underworld. Collectively, these examples highlight how Constella’s commitment to data quality empowers their AI-powered solutions to deliver significant cybersecurity impact.
Google’s Gmail has achieved significant success in the realm of phishing detection by leveraging machine learning to scan billions of emails daily. This software identifies and blocks phishing attempts with a high degree of precision. The system learns from each detected phishing attempt, continuously enhancing its ability to recognize new and evolving phishing techniques. This massive scale of operation and the high accuracy rates demonstrate the power of AI when trained on large volumes of well-labeled, clean, diverse email data.
CrowdStrike and SentinelOne show how AI-enhanced EDR can improve threat detection and response on endpoint devices. (https://www.sentinelone.com/cybersecurity-101/data-and-ai/ai-threat-detection/). AI monitors devices for anomalies and responds in real time to detect, contain, or neutralize potential threats. The effectiveness of these platforms relies on their ability to analyze vast amounts of endpoint data to establish baselines of normal activity and to quickly identify and react to deviations that signify anomalous activity.
Getting Predictive
AI algorithms now play a growing role in analyzing extensive repositories of historical security incident data. These repositories typically include records of past breaches, detailed indicators of compromise (IOCs), and intelligence on known threat actors. By mining this historical information, AI can uncover hidden trends and recurring patterns that manual analysis might easily miss. Provided the data is high quality, machine learning models can then use these patterns to predict the likelihood of specific cyberattacks occurring in the future (https://www.datamation.com/security/ai-in-cybersecurity/). As a result, predictive analytics empowers organizations to adopt a more proactive security posture—strategically reinforcing defenses and allocating resources toward the most probable targets. In essence, predictive analytics stands as a cornerstone capability of AI in cybersecurity, enabling threat anticipation and smarter security prioritization.
Consider an organization that utilizes AI to analyze its comprehensive historical security incident data. AI detects recurring phishing attacks targeting finance and HR before fiscal year-end, revealing a seasonal pattern of threats. The organization uses AI predictions to launch tailored security training for finance and HR ahead of high-risk periods. Training helps employees recognize known phishing tactics and avoid similar attacks during future high-risk periods. AI tracks how attacker techniques evolve over time, going beyond just predicting attack type and timing. Organizations can adapt defenses in advance, using AI insights to counter evolving attacker techniques and future threats. Mastercard, for instance, uses AI for predictive analytics to analyze real-time transactions and block fraudulent activities. IBM’s Watson for Cyber Security analyzes historical data to predict future threats.
Detecting Insider Threats and Account Compromises
Organizations increasingly employ AI-powered User and Entity Behavior Analytics (UEBA) tools to analyze vast amounts of user activity data. These include login attempts, file access patterns, network traffic generated by specific users, and their usage of various applications (https://www.ibm.com/think/topics/user-behavior-analytics). The primary goal of this analysis is to establish robust baselines of what constitutes “normal” behavior. This applies to both individual users within the organization and for defined peer groups based on their roles and responsibilities. ML algorithms are then applied to continuously monitor ongoing user activity. The goal is to detect any significant deviations from those established baselines. SThe system flags such deviations as potential signs of compromised accounts, malicious insiders, or other suspicious behavior.
Anomalies may appear when users log in at unusual times or unexpected locations, access sensitive systems outside their usual scope, transfer unusually large volumes of data, or suddenly shift their typical activity patterns. UEBA systems use AI-driven risk scores to rank threats, helping security teams prioritize the most suspicious users and activities. In some cases external sources of identity risk intelligence are factored in as well (https://andresandreu.tech/disinformation-security-identity-risk-intelligence/). UEBA solutions use AI/ML to track user behavior and detect deviations. They also transform raw data into actionable insights by baselining normal behavior and detecting anomalies from there.
Consider an employee who consistently logs into an organization’s network from their office location during standard business hours. An AI-powered UEBA system has this user flagged as risky. This is based on an identity risk posture score that shows evidence of infostealer infections. The UEBA system continuously monitors relevant login activity. It detects a sudden login attempt originating from an IP address in a foreign country at 03:00, a time when the employee is not typically working. This unusual login is immediately followed by a series of access requests to sensitive files and directories. The employee in question does not normally interact with these files as part of their job responsibilities.
The AI system, which already has the user account flagged as risky, recognizes this sequence of events as a significant deviation from the employee’s established baseline behavior. In turn, it flags the activity as a high-risk anomaly. This strongly indicates a potential account compromise and promptly generates an alert for the security team to initiate an immediate action. Beyond detecting overt signs of compromise, AI in UEBA can also identify more subtle indicators of insider threats. For example, attackers may exfiltrate data slowly over time—a tactic that traditional security tools can easily overlook.
AI-driven UEBA needs clean, consistent data from logs, apps, and network activity to build accurate behavioral baselines. Poor data—like missing logs or bad timestamps—can cause false alerts or let real threats go undetected. AI must learn user-specific behavior and adapt to legitimate changes like travel or role shifts to reduce false alarms. Organizations must protect user data and comply with regulations when using systems that monitor and analyze behavior. Finally, it is important to be aware of potential biases that might exist within the user data itself. Biases may cause AI to unfairly flag certain users or behaviors as suspicious, even when they’re actually legitimate.
Part 8 will conclude this series and cover some unique data quality challenges in the cybersecurity domain. Data quality is the foundation for data-powered AI: proven cybersecurity examples you need to see.