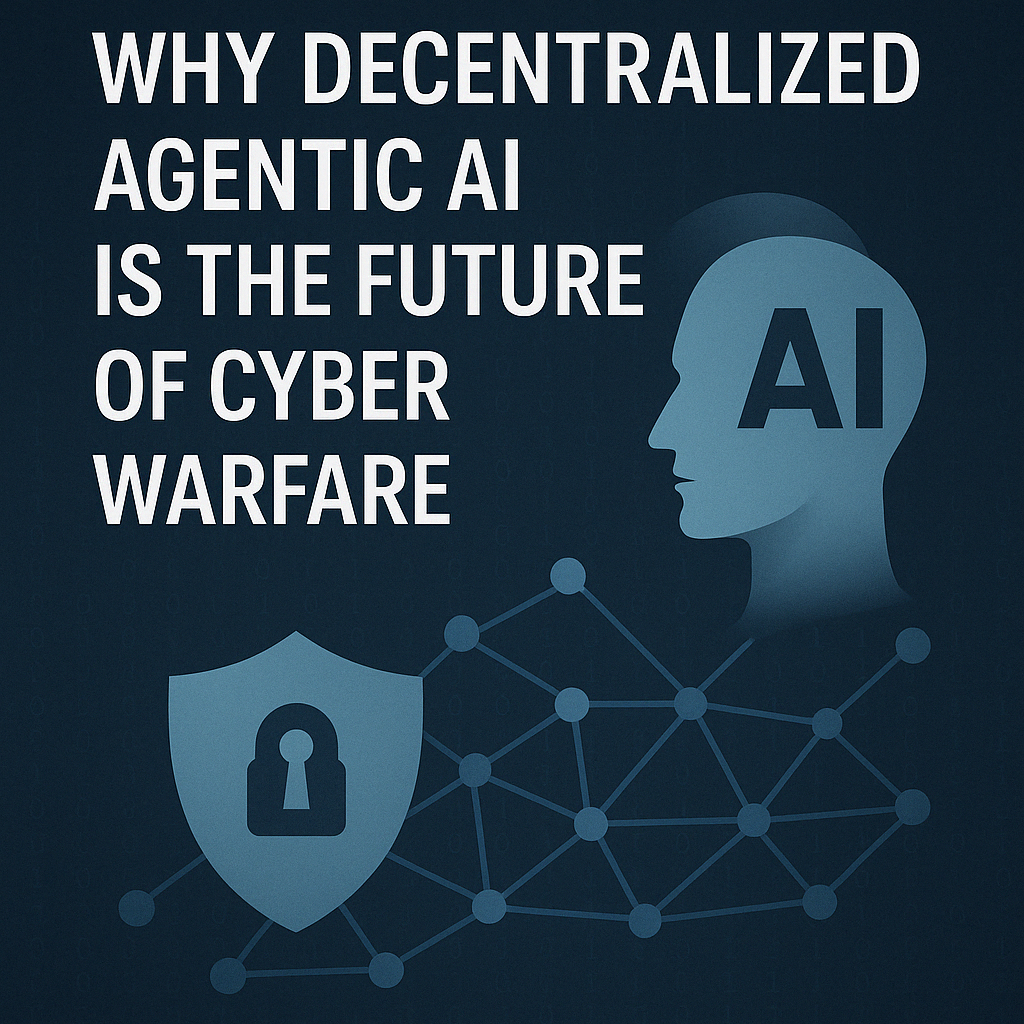
Agentic Artificial Intelligence (AI) (What Is Agentic AI?) is becoming a powerful force in cybersecurity and modern warfare. These AI systems consist of autonomous agents with minimal human oversight. They perceive, decide, and act independently to achieve specific goals. Both defenders and attackers now wield unprecedented digital power. These agents can write code, hunt threats, and execute complex operations. One analyst called agentic AI a “huge force multiplier” for cybersecurity teams (Agentic AI is both boon and bane for security pros). At the same time, attackers can use it to craft phishing lures and create advanced malware. This dual-use nature makes agentic AI a double-edged sword in cybersecurity. That’s why decentralized agentic AI is the future of cyber warfare.
In the military domain, the consequences are even more severe. Cheap AI-powered drone swarms could threaten advanced weapons and shift the global balance of power. Decentralized, autonomous agents are transforming cyber and kinetic warfare. This emerging ecosystem evolves faster than we can control it. Experts predict attackers will exploit vulnerabilities in half the time it takes today.
What is Agentic AI?
Agentic AI refers to AI systems that can act as independent agents, pursuing goals through sequences of actions in a given environment. Traditional AI stops after output. These systems often consist of multiple specialized agents working together. Each agent might handle a subtask (e.g. monitoring logs, scanning for vulnerabilities, or controlling a drone). Together they orchestrate complex workflows to achieve an overall objective. In other words, agentic AI extends generative or analytical AI models by giving them a type of freedom. This latitude enables the capacity to make decisions and take actions without constant human prompts.
A key feature is that agentic AI can maintain long-term goals and react to real-time conditions. An agent might continuously monitor a web application’s state. It reasons about potential threats in real time. The agent can take actions like updating a Web Application Firewall (WAF) dynamically. Agents use reinforcement learning and planning algorithms to choose optimal responses. They often integrate Large Language Models (LLMs) for perception and reasoning. Other Machine Learning (ML) models may also support their decision-making. Agents are not static systems. They are designed to learn from experience and adapt over time. Agentic AI takes things further by coordinating groups of agents through custom integrations. This gives the agents greater contextual awareness and the ability to act in concert.
Varying agentic architectures exist. The design of the architecture must be tailored to the problem being solved. Some are hierarchical with a “conductor” agent overseeing multiple subordinate agents. This vertical design can be effective for linear workflows, but it introduces a single point of control that could become a bottleneck. Other architectures are more horizontal, with agents working as peers in a distributed fashion. In such a decentralized design, there is no single leader. Disparate agents collaborate or even compete, sharing information and dividing tasks among themselves. This latter approach is often slower to converge on a solution than a tightly managed hierarchy. But, it introduces major advantages in its ability to scale as well as its level of resilience and adaptability.
Decentralized Agents and Swarm Intelligence
Decentralization makes agentic AI very powerful because it removes the reliance on any central coordinator. Moreover, it enables swarm intelligence. Swarm intelligence draws inspiration from ant colonies and bee hives. It drives how simple agents follow rules and interact with each other (Military Drone Swarm Intelligence Explained). In a decentralized AI system, each agent makes decisions based on the combination of its own observations and signals from its peers. In this mode of operation there is no waiting for commands from a top-down, central controller. Each individual agent is not capable of anything earth shattering. But numerous agents working in unison can solve problems no single agent could handle alone.
Swarm AI
Swarm AI has been introduced into the cybersecurity space to leverage the swarm concept. It involves deploying autonomous agents across an ecosystem in a mesh formation, where each agent (or node) can process data and share relevant insights peer-to-peer (What is Swarm AI and How Can It Advance Cybersecurity?). A key benefit to this technology is the real-time collective learning and response. If one agent detects a threat, it can immediately broadcast that to its peers. This allows the entire swarm to adapt in almost real-time. This stands in contrast to traditional centralized systems that might suffer lag or single points of failure in communication.
Some of the advantages of decentralized swarms include:
- No single point of failure – agents can act individually or collectively with no central server. This makes for a robust system. If one node fails, others quickly adjust and continue operations. The notion of self-healing becomes real and there is resilience to attacks or failure within swarms.
- Scalability and coverage – a swarm can expand past the boundaries of traditional networks, with each agent handling local data. This scales naturally, with a swarm being able to dynamically add more agents to increase coverage and/or processing power.
- Real-Time responsiveness – each agent reacts to local conditions relative to encountering them, without needing approval from a central brain. For example, a device-level agent can quarantine a malware outbreak on a single host, while simultaneously informing others to be on the alert.
- Adaptability and learning – decentralized agents share observations to collectively refine their larger strategies. The swarm as a whole can continuously adapt and learn by distributing new knowledge to all swarm members. If one agent discovers a novel attack vector, all agents can update their detection models in concert.
- Privacy and trust – by processing data locally agents can limit what gets shared with swarm peers. This decentralized approach can protect sensitive data better than centralizing all raw data. Developers use blockchain-based communication to let agents trust each other’s signals without revealing private data. A project called Naoris Protocol, for instance, employs a blockchain-backed swarm of cybersecurity agents to share threat intelligence across organizations securely in a decentralized mesh.
Cyber attacks often start from many points and spread across systems, like in botnets or Distributed Denial of Service (DDoS) attacks. Deploying a distributed defense matches this structure and makes strategic sense. Compounding the effectiveness factor, the lack of a central command makes a decentralized system harder to predict or defeat. Adversaries cannot simply “cut the head off the snake” as there is no head at all.This was illustrated in a U.S. Department of Defense (DoD) test where a swarm of 103 Perdix micro-drones was launched from fighter jets. The drones organized themselves via a swarm pattern, reforming their flight trajectories on the fly without any single drone leading (Meet the future weapon of mass destruction, the drone swarm). In essence, this is a parallel to a decentralized swarm that contributes to a collective intelligence that can outperform a monolithic AI agent on complex, enterprise level problems.
Defensive Applications of Decentralized Agentic AI
Decentralized agentic AI offers powerful new defensive capabilities in cybersecurity. Security teams can deploy swarms of intelligent agents to act as always-on, adaptive sensors operating at varying parts of a network. These autonomous defenders can monitor systems continuously, do so at different levels (e.g. endpoints, network, industrial devices, etc), detect threats faster than humans, and even coordinate automated responses across an enterprise. All of that can take place without requiring human direction.
Intrusion Detection
One interesting use case is real-time intrusion detection. But this model of operation can also include responses. Instead of a single security solution inspecting traffic, imagine a fleet of lightweight AI agents on every endpoint and subnet, all collaborating in close to real time. Each agent analyzes local events (e.g. network packets, login attempts, file changes, etc) and shares alerts or anomalies with the entire swarm. This makes possible a distributed Intrusion Detection System (IDS) where suspicious activity is detected and acted upon in seconds.
Swarm-based IDS agents can identify abnormal conditions and propagate relevant data to peers, who then collectively can decide on responses and/or countermeasures. For example, if one agent detects a brute force attack against an Application Programming Interface (API) header that grants access via a key. Peer agents could automatically adjust their Web Application Firewall (WAF) rules across disparate cloud hosting providers. All of that can take place faster than the traditional log shipping to a SIEM and subsequent analysis that typically is necessary.
Threat Hunting
Another area of interest is autonomous threat hunting. Agentic AI “hunters” can proactively sweep through logs, user behavior, and system telemetry 24/7 in search of hidden indicators or signals. These agents can also use ML to find patterns humans might miss across large volumes of data. Because they operate in parallel across the environment, they can cover a huge range of hypotheses quickly. If one agent uncovers a signal (e.g. unusual privilege escalation), it can enlist others to follow in pursuit and cover much ground in divide and conquer style.
This type of adaptive hunting has the potential to catch advanced threats that evade signature-based tools (Agentic AI: How It Works and 7 Real-World Use Cases). It also reduces fatigue on human analysts by filtering out false positives and handling routine tasks. In fact, autonomous agent platforms are surfacing that automate alert triage and Security Operations Center (SOC) routines that were once manual. This frees up human analysts to focus on confirmed alerts and/or incidents (Agentic AI and the Cyber Arms Race).
Incident Response
Crucially, decentralized defense agents can also coordinate active responses to incidents. These are more akin to real time countermeasures than the traditional incident response world of playbooks and system recovery. As an example, North Atlantic Treaty Organization (NATO) researchers have outlined an architecture for Autonomous Intelligent Cyber Defense Agents (AICA) (https://ccdcoe.org/uploads/2018/11/Towards_NATO_AICA.pdf). These would essentially be cyber hunter-killer agents deployed in military networks.
According to a NATO report, friendly cyber agents will work in swarms to detect cyber-attacks, devise countermeasures, and adapt their response. The vision is that these defensive swarms would stealthily patrol networks, find and fight nefarious activity in real-time without waiting for human instructions. NATO experts argue that only collective intelligence from swarms of agents would be effective against a sophisticated, coordinated cyberattack, especially in a military setting. Notably, the NATO study warns that “without active autonomous agents, a NATO C4ISR network will not survive an encounter with a determined, technically sophisticated enemy”.
Beyond theory, there is evidence of defensive agentic AI in practice:
- Copilot agents – there have been demonstrations where agents autonomously talk to disparate security products (e.g. SIEM, endpoint, identity systems) to identify vulnerabilities and compromised assets in an enterprise environment (https://www.microsoft.com/en-us/security/blog/2025/03/24/microsoft-unveils-microsoft-security-copilot-agents-and-new-protections-for-ai/). Essentially, each agent is specialized (one might watch identity systems, another cloud configs, etc.) and the Copilot orchestrates their findings. This is an example of multiple agents coordinating to improve a defensive posture.
- Autonomous penetration testing – running red team agents is a defensive tactic to find weaknesses before real adversaries do. Agentic AI can simulate realistic multi-stage attacks against an organization’s own systems continuously. Unlike human-led pen-tests that happen periodically, autonomous agents can hammer away at defenses continuously. By employing such agentic “attack” bots in a controlled way, defenders can expose weaknesses and harden their systems faster. This is decentralization at another level, instead of one small team of human red-teamers, one can have hundreds of relentless AI agents probing environments in parallel.
- Security orchestration – Agentic AI is also improving how SOCs function internally. Agents can automate the handling of incidents and related steps (e.g. opening tickets, documenting steps, sending communications, etc). For instance, one agent detects a malware outbreak and isolates impacted hosts, then signals another agent to gather forensic data or notify admins. This kind of automation at scale means incidents get contained and resolved with minimal human delay.
Ultimately, decentralized agentic AI gives defenders the possibility of speed, scale, and adaptability that traditional tools simply cannot match. By distributing intelligent agents throughout networks and systems, living, intelligent, cooperative defensive mechanisms are possible. These mechanisms come with the promise of observability and action everywhere at once. Early results are promising, but defenders must also prepare for the flip side as attackers have access to the same technology.
Offensive Implications: Decentralized AI as a Threat
Unfortunately, the power of decentralized agentic AI makes it a double-edged sword. The same capabilities that benefit defenders can be harnessed by malicious actors to create more sophisticated and possibly even resilient cyber attacks. To an extent this is the beginning of the era where AI-driven threats operate in a decentralized, swarm-like manner and they will overwhelm traditional defense mechanisms.
Malware
One area of concern is that of swarm malware. This is essentially a network of AI-powered malicious agents that collaborate like a team of attackers, without a central command server (Swarm Malware: How AI-Powered Attacks Are Redefining Cyber Warfare). Traditional botnets usually rely on a Command-and-Control (C2) server and follow pre-programmed instructions. In contrast, a swarm malware attack involves adaptable independent malware instances that communicate peer-to-peer, make intelligent decisions (e.g. reinforcement learning), can act in polymorphic form, and even self-modify to evade detection.
For example, one infiltrated agent might quietly map out a network’s topology and hunt for points of ingress; if it finds something of interest, it can signal the rest of the swarm which then converge to exploit that target. All the while another subset of bots work to disable security logging. All of this can happen very rapidly. We have already encountered this level of sophistication with some Advanced Persistent Threat (APT) cases; this simply exaggerates the threat due to the distributed nature, possible speed of attack, and the necessary level of coordination.
Some of the features of AI-driven swarm attacks that make them especially interesting are:
- Peer-to-Peer coordination – swarm bots communicate over decentralized channels like encrypted P2P networks, blockchain transactions, or anonymous networks (e.g. Tor). This means there is no single C2 server for defenders to find and take down; the instructions are coming from within the swarm itself. For example, agents can publish and read commands on a blockchain, which is very hard to block. If defenders find and remove some agents, the remaining ones detect the change and reroute communications. They might switch to DNS or SSH tunneling to adapt and maintain swarm cohesion.
- Autonomous decision making – each malicious agent can generally mimic thinking for itself using AI algorithms. Reinforcement learning allows the malware to improve across multiple iterations, learning what techniques work or don’t work against a specific set of targets. The agents don’t need to wait for instructions; they can be coded to evolve their attack strategies in real-time. They might even go polymorphic, mutating their payloads on the fly to avoid antivirus detection. This autonomy makes them unpredictable and pattern matching becomes of less utility in these scenarios. A swarm can also exhibit emergent attack behaviors that its creators may not have explicitly programmed.
- Specialization and multi-vector attacks – just as defenders can use specialized agents, attackers can assign roles to different AI agents in a swarm. For example, an agent can be programmed to perform reconnaissance, another one can be focused on exploit execution, there can be evasion focused agents to cover tracks, and there can be mutation agents to ensure a pattern is never exposed. Working together, these agents can create a problematic scenario for defenders. This can become overwhelming for most environments in their current state. It’s the digital equivalent of a wolf pack hunting prey, some distract the sentries, others go in for the kill.
Evasion
Realistically, decentralized malicious swarms are hard to detect and contain. Traditional security tools that look for centralized C2 traffic or known malware signatures struggle against a shape-shifting, adaptively communicating swarm. Law enforcement finds it difficult to shut down infrastructure when the “infrastructure” is a non-static hive of agents coordinating over standard protocols. Instead of noisy obvious attacks, AI agents enable stealthy penetration of a specific target. For instance, an agentic malware could infiltrate an enterprise. Then it can patiently analyze the internal network to find the most valuable data or the keys to escalate privileges. Cooperating AI agents can now do in hours what once took skilled hackers weeks of manual effort. These agents don’t take sick days or face personal issues, enabling nonstop operations.
There is already an uptick in AI-enhanced cyber attacks. Real breaches are basically getting assistance from AI. For example, the 2022 Activision breach was enabled by a series of convincing AI-generated phishing texts that tricked an employee. These stand to become more problematic over time. Imagine phishing emails not just written by AI, but orchestrated by an agent that monitors social media in real time. Autonomous agents with access to public APIs can learn patterns and strategically schedule communications when the target checks email.
Cyber Arms Race
Strategically, nation-state APTs are also eyeing agentic AI to enhance their campaigns. Given this, the “cyber arms race” is a very real concern. If one nation develops powerful cyber agent capabilities, others will follow suit. In some cases the technology even gets shared. The race is accelerating the co-development of attack and defense in cyberspace. Attack agents get better, so defensive agents retrain to adapt, prompting attackers to create even more advanced techniques, and so on. However, this dynamic could also break the entry barrier and the nation-state notion starts to play a lesser role. Ultimately, this means that launching successful decentralized attacks becomes possible by many more groups than what is current state.
Currently, the most devastating cyber weapons (e.g. Stuxnet) are within reach of only a few well-resourced actors. This is due to the expertise and effort required to use them. Agentic AI might democratize the necessary skillset. Moderately capable AI attack agents will soon spread widely, allowing smaller groups or less advanced nations to cause greater impact. Autonomous agents could perform the laborious steps of a kill-chain (e.g. reconnaissance, vulnerability discovery, etc) far faster and at scale. This lets even a small team mount sophisticated attacks.
Asymmetric Cyber Warfare
Asymmetric cyber warfare is fast becoming part of reality. This is where large powers not only have to fend off other nation-states, but also highly capable cyber swarms launched by hacktivists, terrorist groups, or cybercrime groups. Just as nuclear technology eventually spread beyond the initial superpowers (with profound geopolitical effects), agentic AI tech will not stay confined to the “good guys.” This software will spread, and its development will be decentralized globally. This could possibly compress the timeline of nefarious agentic AI proliferation, meaning defensive measures will likely lag behind the threat.
Unpredictability
A big worry is the unpredictability and speed of AI-driven attacks. The worry is the real possibility of accidental escalation. Autonomous cyber operations happen at machine speed. If a swarm of AI agents targets critical infrastructure, the target might struggle to attribute the source of the attack. This potentially causes confusion or misdirected retaliation. In military scenarios, there’s concern that an AI may take an action that crosses a threshold without explicit human checks and balances, simply because the AI deems such action optimal. This lack of transparency and control is a new kind of risk, an AI-ignited flash conflict. Clearly, the offensive implications of decentralized agentic AI demand that we invest just as heavily in countermeasures and kill switches as we do in the agentic technology itself.
Agentic AI in Military Operations
The influence of agentic AI extends beyond the realm of cybersecurity. It is poised to impact military operations as well. Decentralized AI agents are becoming critical in both the digital domain (espionage, cyber attacks, cyber defense) and the physical domain (autonomous drones, robotic swarms, battlefield management).
Military Kinetic Operations
Emotionally, the most enticing application of agentic AI is in autonomous drone swarms and robotic systems on the battlefield. Militaries worldwide are developing swarms of unmanned systems (aerial drones, ground robots, naval drones). These swarms can perform missions collaboratively with minimal direct human control. Decentralized AI is the brains behind these swarms, enabling them to adapt to battlefield conditions, make split-second decisions, and coordinate maneuvers in cohesive form.
Defense contractor Thales recently demonstrated a system called COHESION for drone swarms with high autonomy (Thales demonstrates its capacity to deploy drone swarms with unparalleled levels of autonomy using AI). In tests, swarms of drones were able to carry out missions even under conditions where Global Positioning System (GPS) and other communications were jammed. This success was only possible because the drones could perceive their local environment, share information amongst each other, and collaboratively adjust tactics without needing continuous human commands. The drones identified targets, analyzed enemy movements, and reprioritized their objectives on the fly. In doing so they effectively accelerated the military Observe, Orient, Decide, Act (OODA) loop for faster decision-making in combat situations.
Importantly, these swarm systems aim to reduce the cognitive load on human operators. Theoretically, one operator can supervise an entire swarm rather than manually flying a single drone. This force multiplication means militaries can deploy dozens or hundreds of assets with the manpower that typically control one asset.
The strategic implications of drone swarms are enormous. Advanced militaries have invested in expensive platforms (e.g. aircraft carriers, stealth jets, etc). These investments assume they won’t face swarms of inexpensive kamikaze drones capable of overwhelming the defenses they have acquired. That assumption is no longer safe. Insurgent groups, hactivist groups, and mid-tier nations can afford low cost drones that can have explosives attached to them. With AI swarm technology, these typically underwhelming forces could coordinate an attack where dozens of drones simultaneously dive onto a warship or a tank battalion, overwhelming its defense systems.
In April 2025, a U.S. CENTCOM commander stated that drones are among the top threats faced by forces, and swarms are an even bigger concern than individual UAVs (https://cuashub.com/en/content/centcom-colonel-discusses-the-challenge-of-adapting-to-the-drone-threat/). Imagine, a swarm of drones that cost $1,000 USD could potentially destroy a warship that cost $1 BN USD. To respond, entities such as the U.S. DoD are not only seeking anti-swarm defenses (like directed-energy weapons), but also building swarms of their own. As of 2020, the DoD had multiple programs and contracts explicitly focused on AI-coordinated drone swarms, recognizing that whoever masters swarming gains a tactical edge.
Military Logistics
Beyond battlefield drone operations, multi-agent AI is improving military logistics and planning. Agentic AI can effectively coordinate supply convoys, allocate tasks to autonomous robotic vehicles, and manage battlefield communications dynamically. This last point is important because agents could have visibility into areas where humans may not. In strategic planning, the U.S. DofD is exploring agentic AI to support war-gaming and operational planning. The implications are grand as agents can synthesize vast amounts of intelligence and generate unbiased decisions much faster than human staff alone (AI’s New Frontier in War Planning: How AI Agents Can Revolutionize Military Decision-Making).
An agentic AI could become a powerful advisor, analyzing geopolitical data, battlefield intel, and logistics in parallel to propose optimal strategies. By integrating such AI into command centers, commanders might get decision options in minutes that would take weeks via manual planning. This speeds up the command decision cycle, crucial in fast-moving conflicts. Agentic AI can become the next big thing in maintaining or gaining decision superiority, this is the ability to observe, decide, and act faster than the adversary.
Agentic AI and decentralization are driving a new era of warfare. This is one where swarms of autonomous agents, whether in cyberspace or the physical world, confront and engage each other. Warfighters may increasingly find themselves orchestrating AI teammates while countering enemy AI. This new era comes with many challenges around trust, rules of engagement, and control, but militaries cannot ignore these technologies now.
Challenges and Safeguards
While the potential of decentralized agentic AI is immense, it does come with significant challenges, risks, and ethical considerations:
- Reliability and control – by design, agentic AI reduces direct human control. This autonomy means agents might make mistakes or take unexpected actions. For example, a defensive agent could mistakenly shut down a critical server thinking it contains malware. In essence this creates a self-inflicted denial of service. In military use, the stakes are higher – what if a drone swarm interprets a civilian convoy as hostile due to faulty signals? Ensuring robust guardrails is essential. Industry recommendations include having configurable thresholds where an AI must pause and get human approval if an action crosses a certain threshold.
- Accountability and ethics – when an autonomous agent causes damage, who is responsible? This is a dicey issue. Legal and ethical frameworks lag behind in the area. We currently treat software as tools under human responsibility, but truly autonomous agents blur that line a bit. In military scenarios, deploying lethal autonomous agents raises obvious ethical questions. International discussions have begun around potential treaties or at least guidelines for lethal autonomous weapons, often focusing on keeping meaningful human control. Meanwhile, organizations using agentic AI for security must implement governance policies that can be enforced.
- Security of the agents themselves – ironically, the AI agents we deploy for defense could become targets of attack. This is seen in parallel today where products that are supposed to protect an environment get broken into themselves. Adversaries will try to trick or subvert defensive AI agents. Multi-agent systems also introduce new elements of an attack surface. If agents communicate peer-to-peer, could an attacker inject a rogue agent into the swarm to feed false information or disrupt coordination? Researchers have noted the possibility of poisoning attacks on cooperative multi-agent systems, where manipulating one agent’s behavior can degrade the performance of the whole team (One4All: Manipulate one agent to poison the cooperative multi-agent reinforcement learning). Strong inter-agent authentication, consensus protocols for decisions, and systemic isolation (so one compromised node doesn’t doom the rest) are active areas of research to ensure trust in decentralized AI networks.
- Data privacy and abuse – decentralized agents often need broad access to data (e.g. endpoint data, log files, etc) to be effective. Without proper controls, this raises privacy concerns. Imagine an agent that scans employee communications to detect insider threats; it could inadvertently violate privacy laws or company policies if not carefully configured. Agents need to be coded such that on-device processing means data stays local and only alerts leave the source. The abuse potential of agentic AI is high. There is a responsibility for researchers and vendors to ensure that advances in agentic AI come with corresponding improvements in security and access control.
Despite these challenges, the trajectory is clear. Decentralized agentic AI will play an ever-growing role in cybersecurity and military theaters. To harness its benefits while managing risks, collaboration between AI researchers, cybersecurity experts, and policymakers is vital. Efforts like the Cloud Security Alliance (CSA) guidelines on agentic AI threat modeling (Agentic AI Threat Modeling Framework: MAESTRO) are steps in the right direction. Organizations adopting agentic AI should start with small steps, supervised deployments (e.g. agents that make recommendations, not final actions). This way it is possible to introduce incremental controls that should lead to trust and understanding of the behavior. We cannot afford to make the traditional cybersecurity mistake of it being an afterthought to some deployment. Over time, as confidence and safety mechanisms improve, we can transition more decision authority to these agents.
Conclusion
Decentralized agentic AI represents a major advancement for both cybersecurity and military operations. By empowering networks of autonomous agents to act in concert, we gain systems that are faster, more scalable, and more resilient than traditional centralized approaches. In cyber defense, this means security that can operate at machine speed across an entire organization, swarming to address threats the moment they arise. In warfare, it means smaller, smarter forces wielding swarms of potentially lethal drones or algorithms that can outmaneuver larger traditional forces. The offensive implications are equally powerful. Well-coordinated AI agents can mount sophisticated attacks that challenge even the best defenses, forcing a rethinking of how we position and secure critical assets.
Ultimately, agentic AI is a classic red / blue dichotomy. It will be a force for both offense and defense. As cybersecurity professionals, our task is to stay ahead of the curve as best as possible. Innovations in defensive agentic AI may make this possible. Attackers are innovating on the offense, and we must put proper and equally powerful safeguards in place. Decentralization is a force multiplier, hard stop. It makes AI systems more powerful by leveraging the strength of many. But, it also requires giving up some direct control. With robust design, continuous oversight, and a commitment to ethical use, we can embrace decentralized agentic AI to create more secure and resilient systems. The age of autonomous agents is exciting and here, decentralized agentic AI is the future of cyber warfare. How we navigate its opportunities and risks will define the security landscape of the coming decades.